Electronic monitoring is an important tool supporting fishery sustainability. However, implemented at scale, the amount of video data requiring handling, storage, and review is significant. A tuna longline vessel can produce as much as 6,048 hours of video per year (about 5,500 GB of data). With approximately 150 vessels, this puts the annual data volume for the Hawaii longline fleet at over 800TB of data. This could cost about $20,000 USD just for the hard drives. Longline fishing trips include long periods of inactivity (periods where no fishing occurs) as vessels wait for set lines to hook target catch or travel vast distances alongside highly migratory tuna schools. For artificial intelligence, this presented an opportunity where algorithms could be used to eliminate footage without fishing activity and thus not requiring electronic monitoring review.
We compiled electronic monitoring data from 34 Hawaii longline trips. A team of experts from the Hawaii fishery donated time to support manual annotation of fish and humans within the video data. In total, the project created about 200,000 annotations. Machine learning algorithms were used to delineate fishing activity from other video (non-fishing activity). Segments of non-fishing activity could then be reduced in resolution or deleted entirely to reduce storage and review time.

User interface displaying pre-processing. The pre-processor is searching for catch events amongst a large portion of non-fishing activity video.
Pre-processing raw video can reduce review and storage requirements by upwards of 75%.
Artificial intelligence can improve reviewer workflows if well-designed. Intentional and informed design is required to apply the technology beneficially. There are many opportunities to use AI to support electronic monitoring that don't involve pushing the technology beyond its current capabilities or using sophisticated techniques.
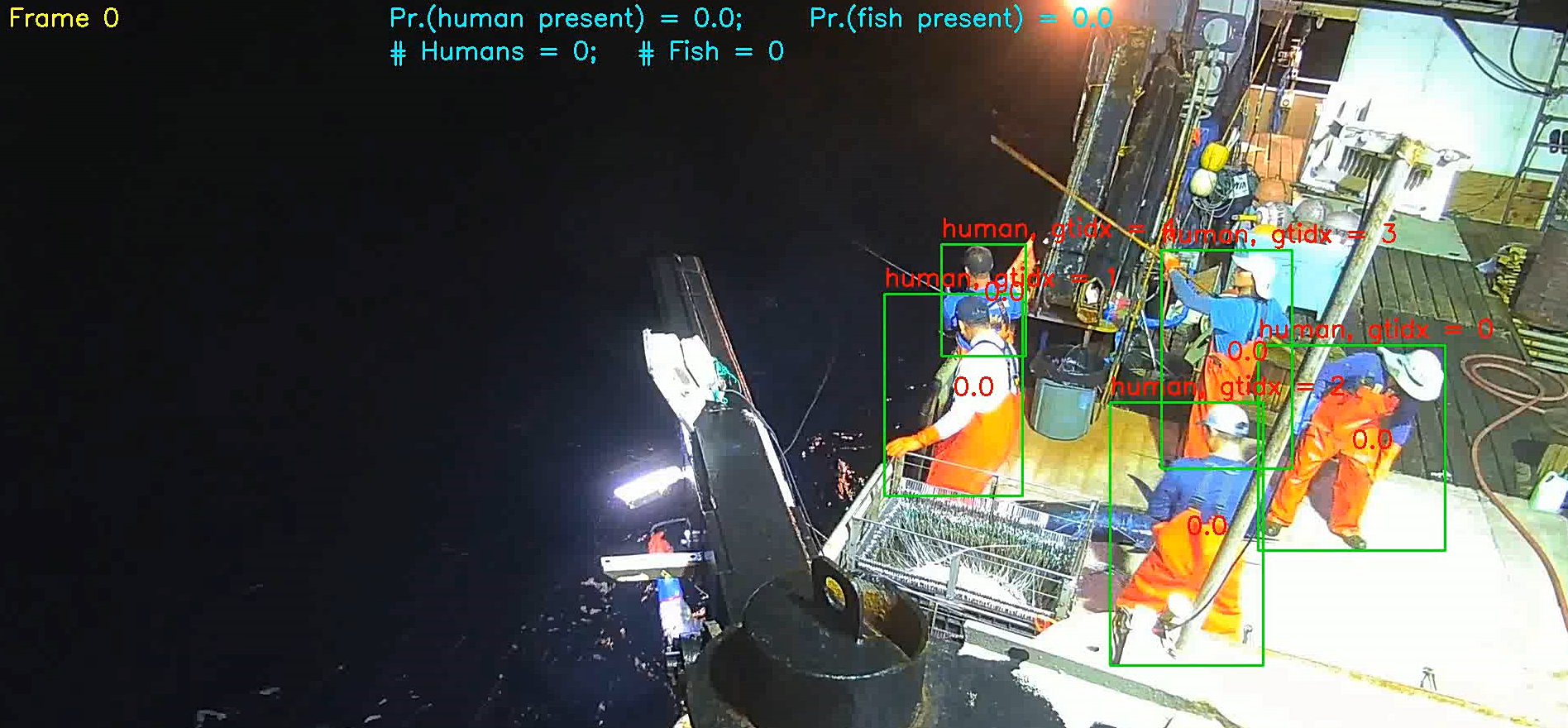
.png)
Designing interfaces around AI rather than adding AI on top of existing offerings without full consideration can lead to great improvements in existing review processes, time, and cost. A significant opportunity exists to support widespread adoption of electronic monitoring through AI-assisted EM review.